
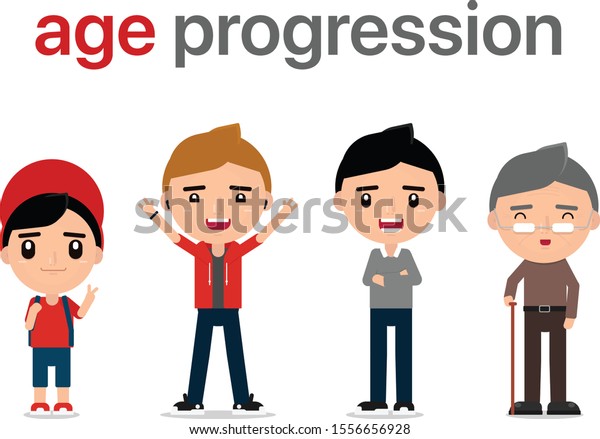
It is indicated by face geometry, skin texture, skin color, etc. Face age synthesis or face age progression is a human face appearance at different ages though preserving their unique identity. An abundance of research is present to resolve these practical issues. However, face age progression remains a challenging task because of facial variations like illumination effects, many intrinsic and extrinsic effects on the face.

Because it is unique for each individual and provides a guarantee of accuracy and security. Nowadays, the face of humans is the most leading biometric area. Further, the use of a biometric system is to identify a person based on some particular characteristics. For an instance, in law enforcement where the face age progression helps to find the missing children or missing person with the help of their previous photo, in face recognition, and in facial analysis like e-commerce platforms, etc. The paper focuses on the various practical applications of super-resolution face aging using Generative Adversarial Networks (GANs).įace age progression is becoming a widely used technique in the modern era as it serves numerous applications. The proposed work is evaluated with quantitative and qualitative methods, produces synthesized face aged images with a 0.001% error rate, and is also evaluated with the comparison to prior methods. Moreover, the experimental results are obtained from five publicly available datasets: UTKFace, CACD, FGNET, IMDB-WIKI, and CelebA. Thus, presents more detailed information in an image because of its high quality. Then image sharpening with edge enhancement is done to give high-quality input to SRGAN, which further generates the super-resolution face aged images. Further, the regex filtering process is performed to separates the synthesized face images from the output of AttentionGAN. Then attention mask is multiplied with the corresponding content mask along with an input image to finally achieve the desired results. One subnet for generating multiple attention masks and the other for generating multiple content masks. AttentionGAN uses two separate subnets in a generator. So, to solve the issue, the proposed work focuses on the realistic face aging method using AttentionGAN and SRGAN. Research has been continuing in face aging to handle the challenge to generate aged faces accurately. The existing face age progression approaches have the key problem of unnatural modifications of facial attributes due to insufficient prior knowledge of input images and nearly visual artifacts in the generated output. In today’s world that demands more security and a touchless unique identification system, face aging attains tremendous attention.
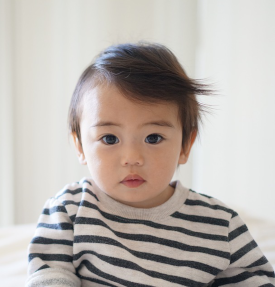
Face age progression, goals to alter the individual’s face from a given face image to predict the future appearance of that image.
